
FaceReader and different scientific theories on emotion
In this blog post, Tess den Uyl (PhD), Peter Lewinksi (PhD), and Amogh Gudi (PhD) from VicarVision outline how FaceReader is designed with scientific rigor and in accordance with responsible AI principles.
FaceReader and different scientific theories on emotion
Emotion has been a popular research topic for decades. This is evident by the vast amount of papers on the topic and the scientific debate surrounding it. For example, a well-known discussion is about the existence of a basic set of emotional expressions and whether emotions are universally similar or culturally diverse1.
There is currently still not a singular theory on emotion that is widely accepted. Some critics also put doubt on the general idea of classification of emotion based on facial expressions2. We understand this leads to questions about the scientific foundation of FaceReader.
In this post, we outline how FaceReader is designed with scientific rigor and in accordance with responsible AI principles, which allows all researchers to use FaceReader within the scientific school of thought that they fit in.
Scientific theories of emotion
There are many different approaches to researching emotions. This is nicely outlined in Figure 1, created by Gross & Barrett (2011)3. Notice the position on the spectrum of the theories of Ekman (red) and Russell (green). They hail from different schools of thought, which are Basic Emotion Theory and Psychological Construction Theory. These main theoretical perspectives have different viewpoint on what constitutes an emotion, what causes an emotion, how emotions are categorized, and how they are researched.
FaceReader offers flexibility to accommodate alternate theories of emotion, enabling users to apply the software within diverse theoretical frameworks and research contexts, thereby broadening its applicability and relevance across various fields of study.

Figure 1. Representative theorists on emotions grouped into 4 major perspectives (from Gross & Barrett, 2011).
Facial expressions and emotional responses
Although these different theories address emotions from a wider perspective, we focus mostly on the relation to facial expressions. Facial expressions are a prominent type of emotional response and the main focus of FaceReader. Many researchers already indicate the relation between facial expression and emotion is not certain, but rather one of probability4. Some researchers focus on the overlapping information and see the universality of emotions (both in individuals and cultures), while others focus on the differences and highlight the diversity within emotional responses.
Currently, there are still researchers theorizing from all these different emotion perspectives. Basic Emotion Theory may be the most openly critiqued, but there are still researchers continuing along the lines of it 56.
In general, the relevance of facial expression research is shown by the large variety of research on emotional expressions7. There is an abundance of research on the recognition and production of facial expressions, and it has been dominated by primary emotions such as fear and happiness. The outputs that FaceReader generates allow the researcher to study emotions from the theoretical perspective they choose.
Build your own interpretation in FaceReader
FaceReader is defined as AFCS (automated facial coding system). FaceReader derives output from the following combinations: facial expressions, eye movements, changes in absorption of light in the face (RPPG), and other auxiliary face parameters. FaceReader can then present that output, arranged in four ways. The first two ways are theory-driven, the third is an agnostic way, and the fourth a user-defined way:
- Basic Emotion Theory (Ekman)
- Circumplex model of emotions with arousal and valence (Russell)
- Facial Action Coding System (FACS) - the most cited agnostic measure of facial expressions
- Custom expressions - together with inputs such as gaze tracking, relative distance to the screen, and RPPG, the user is allowed to build their own output in a GUI*-based module
* A graphical user interface (GUI) is a digital interface in which a user interacts with graphical components such as icons, buttons, and menus.
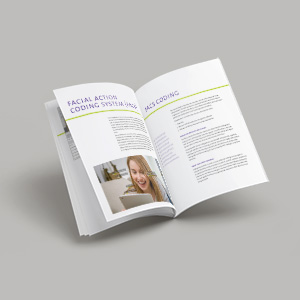
By creating custom expressions, researchers can build their own output. They do this by using existing research on established Action Unit combinations that are associated with different facial expressions of emotions. Or they can choose to create additional dimensions (e.g. approach vs. avoidance based on head position) or physical signals, such as smiling without interpretation labels (for example, 'happiness'). After all, the fact that someone is smiling does not necessarily mean that someone is feeling happy.
User is end-responsible
By constructing FaceReader in a modular way, users can build their own (emotion) classification system. For example, emotion eagerness - how badly does someone wants to buy something - is an emotion closely linked to interest. However, maybe you want to measure something slightly different. In FaceReader, it is possible to build this specific emotion.
The main responsibility is now left to the user as the software provides the three most common ways to interpret the output (basic emotions, circumplex model, and FACS). Also, FaceReader gives a structured drag-and-drop GUI to make one’s own recognition system.
Also read: Creating a custom expression for Engagement: A validation study with FaceReader.
Context is necessary for interpretation
In addition, the study design of experiments allows the researchers to know a lot about the context (e.g., the stimuli or interaction). This helps with the interpretation. Moreover, researchers can perform additional measurements on participants to further shed light on the experienced emotion, such as physiology and eye movements.
FaceReader: from responsible science to responsible AI
There are different approaches to researching emotions. FaceReader allows the researchers to choose the theoretical framework they operate in. This way, FaceReader is built from a responsible science approach, where the FaceReader user completely controls how they will interpret and label the expressions and the signals from their participants’ faces.
Facial expressions are not directly related to the underlying emotional process. Facial expressions are signals, and - like in any measurement tool - there can be a large signal variability. Whether the signal is relevant for a certain process, population, or context should be investigated and validated within each specific research field, using multiple tools.
Furthermore, FaceReader is engineered with Responsible AI principles in mind, striving for privacy-by-design and fair algorithms. For example, it does not use sensitive input (e.g., ethnicity) and it cannot execute facial recognition (i.e., recognizing a person as that specific person). In addition, care is taken to limit bias by gathering training datasets with a balanced representation of gender, age, and ethnicity, and by testing for the degree of bias repeatedly.
In conclusion, the use of different (theoretical) perspectives and a lively discussion are very normal and important in science. FaceReader can be a useful tool to help the field.
References
- See e.g.:
Ekman, P. (1992). An argument for basic emotions. Cognition & emotion, 6(3-4), 169-200. https://doi.org/10.1080/02699939208411068
Gendron, M., Crivelli, C., & Barrett, L.F. (2018). Universality reconsidered: Diversity in making meaning of facial expressions. Current directions in psychological science, 27(4), 211-219. https://doi.org/10.1177/096372141774679
https://www.paulekman.com/blog/darwins-claim-universals-facial-expression-challenged/
Wallbott, H.G. & Scherer, K.R. (1986). How universal and specific is emotional experience? Evidence from 27 countries on five continents. Social science information, 25(4). https://doi.org/10.1177/053901886025004001
Barrett, L.F., Adolphs, R., Marsella, S., Martinez, A. M., & Pollak, S.D. (2019). Emotional expressions reconsidered: Challenges to inferring emotion from human facial movements. Psychological science in the public interest, 20(1), 1-68. https://doi.org/10.1177/1529100619832930
Gross, J. J., & Feldman Barrett, L. (2011). Emotion generation and emotion regulation: One or two depends on your point of view. Emotion review, 3(1), 8-16. https://doi.org/10.1177/1754073910380974
Scarantino, A. (2015). Basic emotions, psychological construction, and the problem of variability. In L.F. Barrett & J.A. Russell (Eds.), The psychological construction of emotion (pp. 334–376). The Guilford Press. https://psycnet.apa.org/record/2014-10678-014
Hutto, D.D., Robertson, I. & Kirchhoff, M.D. (2018). A new, better BET: rescuing and revising basic emotion theory. Frontiers in psychology, 9, 1217. https://www.frontiersin.org/articles/10.3389/fpsyg.2018.01217/full
Keltner, D., Sauter, D., Tracy, J., & Cowen, A. (2019). Emotional expression: Advances in basic emotion theory. Journal of nonverbal behavior, 43(2), 133-160. https://link.springer.com/article/10.1007/s10919-019-00293-3
E.g. as indicated by almost 100k papers on facial+expression+emotion on Scopus and ±25k papers on facial+expression+emotion+fear/happiness/anger
Get the latest blog posts delivered to your inbox - every 15th of the month
more
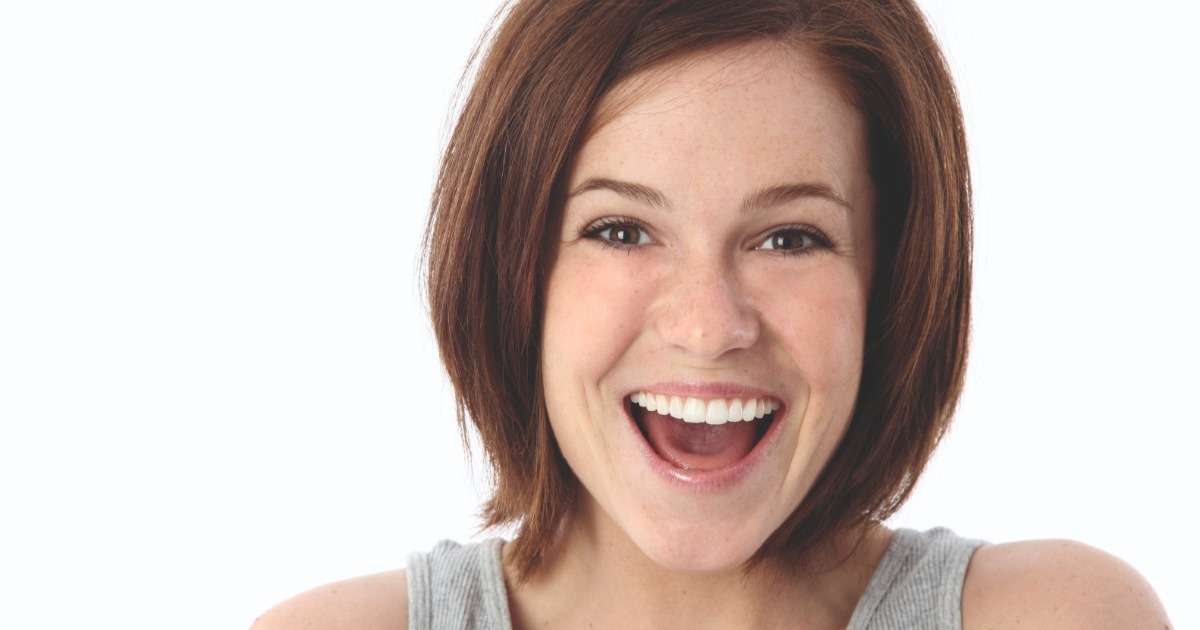
How to use FaceReader in the lab
Are you interested in using automatic facial expression analysis in a standardized lab setting? Here are 5 tips to get you started!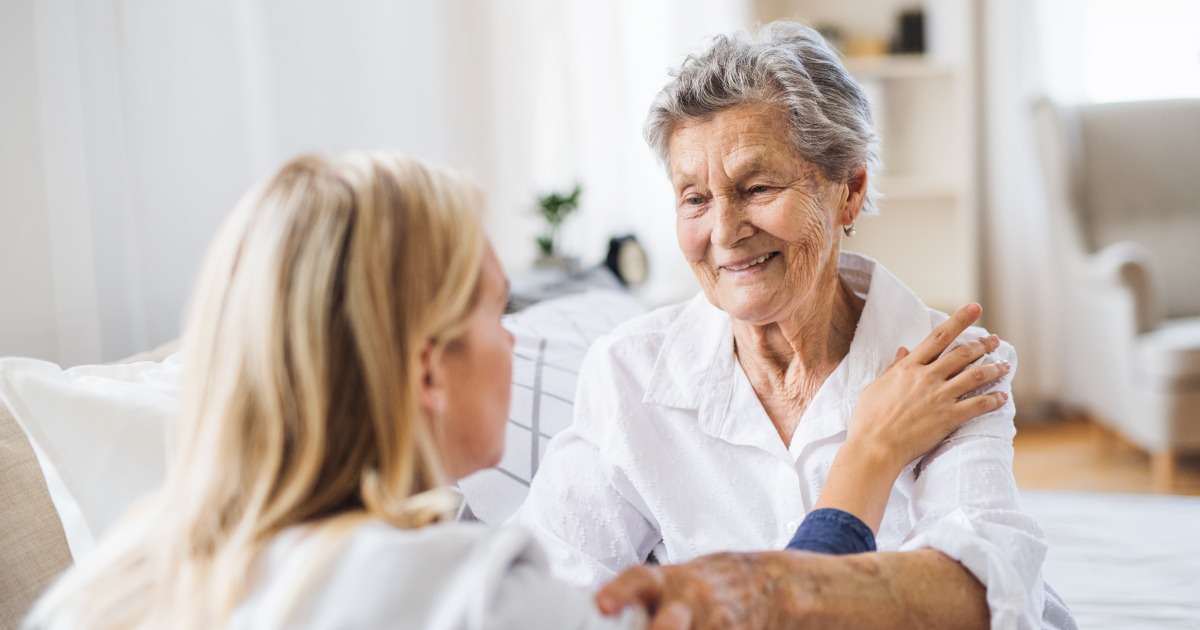
How do people with Parkinson's disease express emotions?
A mask-like facial expression is an early symptom of Parkinson's disease. How can we understand the relation between changed facial expressions and disease severity? Researcher Yang and their team used FaceReader to find out.