
The 5 best ways to use FaceReader: a systematic review
Facial expressions offer a rich source of information about human emotions. Unlike surveys or interviews — which rely on people's ability to articulate what they feel — facial expressions can reveal immediate, unconscious emotional reactions.
One of the areas where facial expression analysis is particularly valuable is consumer behavior research, where emotional reactions can significantly influence decision-making processes. But facial expression analysis is also widely used in other research areas, including behavioral science, medicine, psychology, finance, and education.
In this study1, Elisa Landmann examines the best way to use FaceReader in these different areas of research, focusing on experimental designs, accurate data handling and analysis, and other methodological considerations.
Understanding emotions
In the field of emotion research, two important theories of emotion include the basic emotion theory (Ekman2) and the circumplex model (Russell3).
The basic emotion theory suggests that there are six universal emotions — happiness, surprise, anger, fear, disgust, and sadness — that we express through our facial muscles. These are also referred to as action units (AUs).
The circumplex model describes emotions along a continuum of two main dimensions: valence and arousal. Valence refers to how positive or negative an emotion is, while arousal indicates the level of activity or alertness caused by the emotion.
How FaceReader works
FaceReader is an automated system for facial expression analysis. The software measures facial expressions according to both the basic emotion theory and the circumplex model, providing researchers with a wealth of information. Other benefits of FaceReader include successful validation in different research areas and a very high accuracy rate — validated with the ADFES dataset4.
To learn more about the practical applications of FaceReader in collecting, analyzing, and interpreting emotion data, Landmann performed a systematic literature review. This review includes studies from different areas of behavioral research.
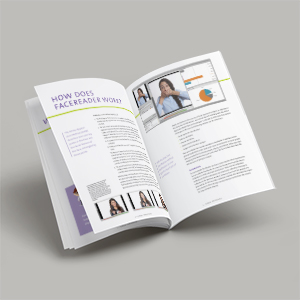
Systematic review of FaceReader
After careful selection, Landmann included 64 FaceReader studies in her systematic review, all from peer-reviewed academic articles published between 2013 and 2023. In her examination, she focused on 5 key areas of research with FaceReader:
- Relevance and benefits
- Collecting and preparing data
- How to handle and analyze output
- Validity of results
- Limiting factors
Below, we will discuss her findings for each of these areas.
Relevance and benefits of FaceReader
FaceReader is used in a variety of research and commercial settings, including medicine, psychology, education, finance, and marketing. As the software is based on algorhitms, facial expression analysis is free from personal bias that would occur in a human coder. It's also much faster.
Dr. Erin Heerey from Western University explains how FaceReader analyzes 800 hours of manual coding in just 14 hours! Read more in this blog post about her research on dyadic interactions.
Landmann also describes FaceReader's benefits compared to other tools on the market, such as classification of individual AUs, a user-friendly interface, having access to data about valence and arousal, and being able to analyze facial expressions in real-time.
Collecting and preparing FaceReader data
To optimize data collection with FaceReader, researchers need high-quality video material with clear visibility of facial expressions. This means focusing on adequate lighting and camera angles, but also instructing participants to look directly into the camera and not move their head too much.
Moreover, FaceReader evaluates the image's or video's quality, helping researchers to gain and select accurate data for their analysis.
By adhering to standardized protocols for data collection and preparation, researchers can enhance the quality and validity of their facial expression analysis.
How to handle FaceReader output
FaceReader provides researchers with both state logs and more detailed logs, which you can export to other (statistical) programs, like Excel or SPSS.
Landmann discusses the different ways that researchers process and analyze their FaceReader data, including AUs and emotion categories. This discussion includes good data handling techniques, such as data cleaning, calculating means or standard deviations, transforming scores into ranks, using cut-off scores, and more.
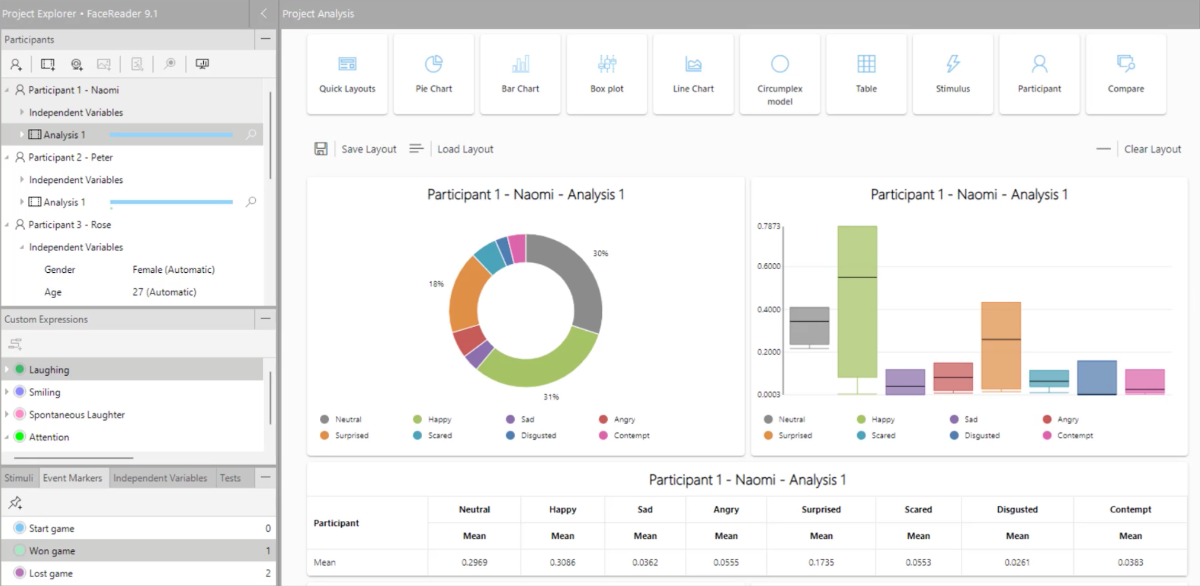
The Project Analysis Module in FaceReader offers advanced analysis of facial expression data. You can easily evaluate stimuli, such as a commercial or an image, by comparing different groups of participants. Create your own filters and base your selection on independent variables.
Validity of FaceReader results
It remains important to evaluate the accuracy and consistency of FaceReader measurements in capturing emotions across different individuals and contexts. For example, by comparing the software's results with established emotion coding systems or human ratings.
Findings from the current literature review show success rates between 80% and 90%, showcasing the software's high accuracy. As Landmann puts it: "FaceReader evaluations do not require any additional correction by humans and even outperform humans in some cases."
Landmann suggests that FaceReader is most accurate when assessing the basic emotions, although the distinction between subtle display of surprise, fear, and anger may need to be overseen by a human coder.
Limiting factors when using FaceReader
Lastly, Landmann discusses the limitations of FaceReader. As the software is only able to analyze one face at a time, she argues that this makes it less suitable to measure emotions of multiple people at the same time. For example, when studying interactions.
Moreover, the quality of an image or video determines whether researchers can use the data — and it can be distorded by factors like using the wrong recording angle or a participant moving too much.
Understanding FaceReader's strengths and limitations is essential for researchers, so they can consider what methods to supplement their facial expression analysis with.
Wide applications of FaceReader
Landmann's study not only highlights the robustness of FaceReader in analyzing emotional responses, but also emphasizes how standardized guidelines help to optimize its use across different research domains.
In marketing, for example, understanding consumers' emotional reactions to advertisements can help improve company content to better meet their needs. In mental health research, objective measures of emotional states could improve the diagnosis and treatment of different disorders.
Landmann suggests that as FaceReader software continues to evolve, its applications will expand, offering deeper insights into human emotions.
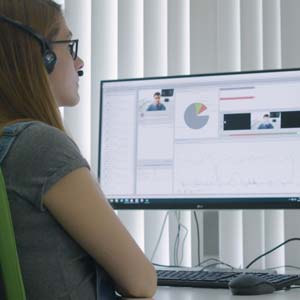
References
- Landmann, E. (2023). I can see how you feel - Methodological considerations and handling of Noldus's FaceReader software for emotion measurement. Technological Forecasting & Societal Change, 197, https://doi.org/10.1016/j.techfore.2023.122889.
- Ekman, P. (1992). An argument for basic emotions. Cognition & emotion, 6(3-4), 169-200. https://doi.org/10.1080/02699939208411068
- Scarantino, A. (2015). Basic emotions, psychological construction, and the problem of variability. In L.F. Barrett & J.A. Russell (Eds.), The psychological construction of emotion (pp. 334–376). The Guilford Press. https://psycnet.apa.org/record/2014-10678-014
- Schalk, J. van der; Hawk, S.T.; Fischer, A.H.; Doosje, B.J. (2011). Moving faces, looking places: The Amsterdam Dynamic Facial Expressions Set (ADFES). Emotion, 11, 907-920. DOI: 10.1037/a0023853
Get the latest blog posts delivered to your inbox - every 15th of the month
more

Why a clear mask is essential for clearer communication
For people who are deaf or hard of hearing, it is essential to be able to see the movements of the mouth while communicating. With the help of clear masks they can access the full facial expressions.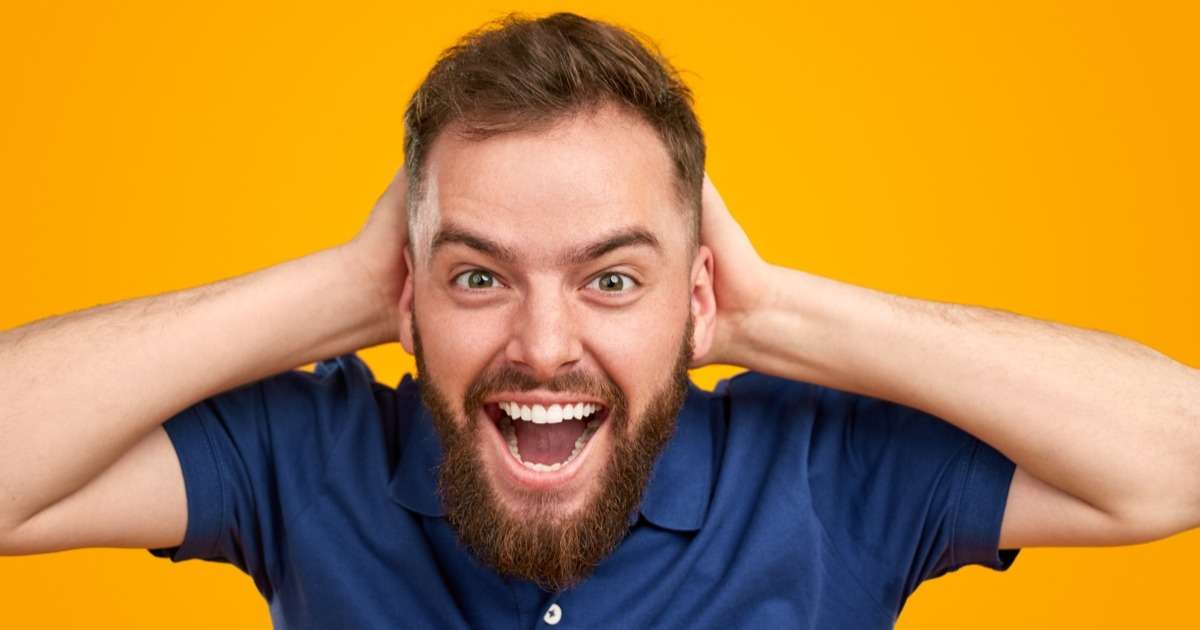
FaceReader and different scientific theories on emotion
In this blog post, Tess den Uyl, PhD, Peter Lewinksi, PhD, and Amogh Gudi, PhD from VicarVision outline how FaceReader is designed with scientific rigor and in accordance with responsible AI principles.