3 easy steps
Kickstart your research
1. Tell us about your research challenge. How can we help?

2. Our dedicated scientific consultants provide you with a tailored solution.

3. With our continuous product developments, we are partners for life.
A selection
Our products

EthoVision XT
EthoVision XT is the most widely applied video tracking software that tracks and analyzes the behavior, movement, and activity of any animal.

DanioVision
Looking for a zebrafish larvae research tool? DanioVision is the complete system designed for high-throughput tracking of zebrafish larvae and other small organisms.
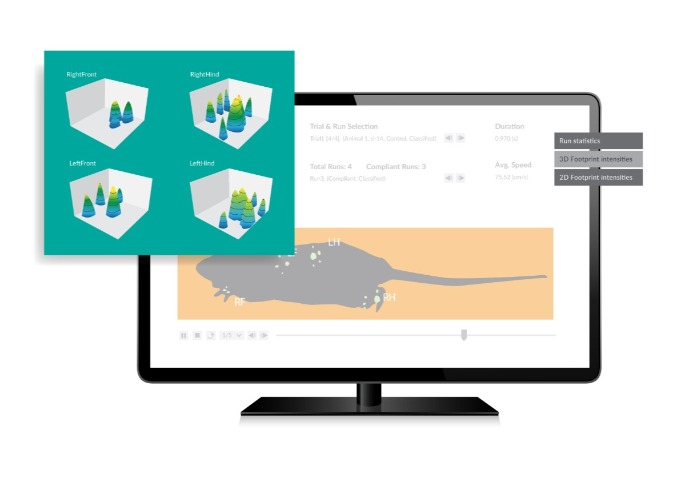
CatWalk XT
CatWalk XT is a complete gait analysis system for quantitative assessment of footfalls and locomotion in rats and mice.
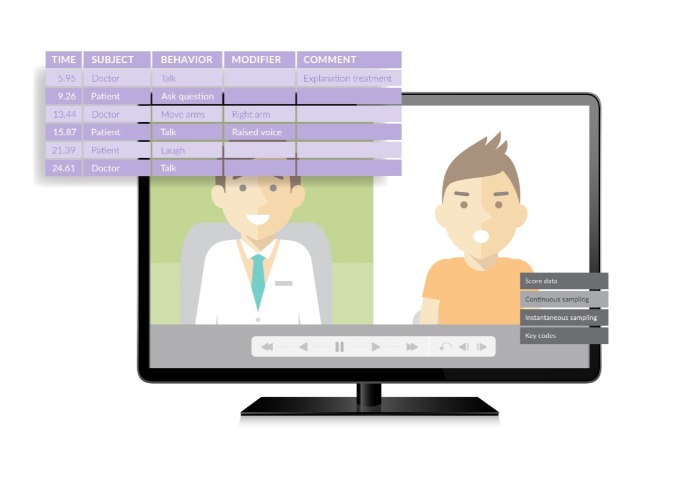
The Observer XT
Supporting you from coding behaviors on a timeline and unraveling the sequence of events to integrating different data modalities in a complete lab.
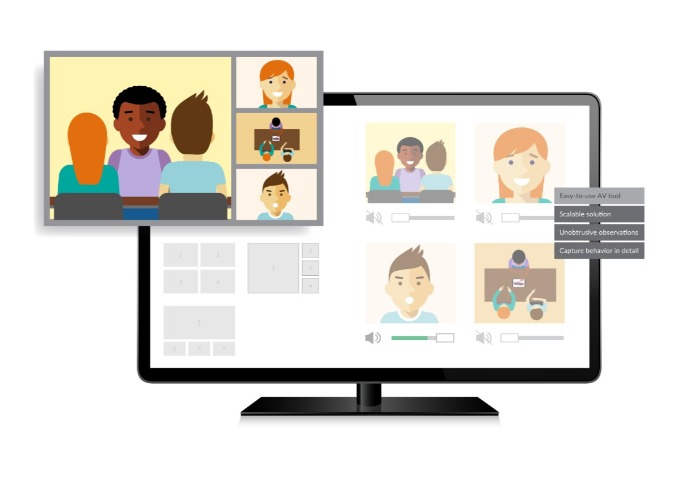
Viso
Viso is the easy-to-use solution for creating video and audio recordings in order to capture behaviors and interactions of your participants.

FaceReader
To gain accurate and reliable data about facial expressions, FaceReader is the most robust automated system that will help you out.
4.5 stars
customer satisfaction
50,000
publications
19 offices
worldwide
60,000 users
around the globe
Around the world
Meet us
Our recent
Research blogs
15
May
Conference
09
Jun
Conference
On-Demand Webinars
Did you miss one of our live talks? Visit our online library to access them all for free.
Or find an overview of all events.
Our recent
Research blogs

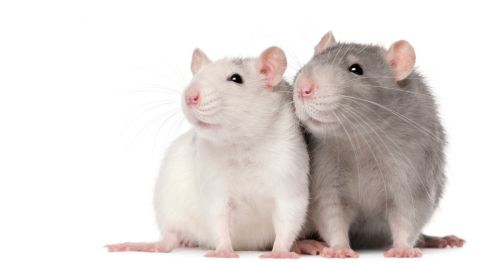
Step into the Future with the NEW PhenoTyper 2 top unit
We have just released our latest hardware innovations: the PhenoTyper 2 top unit. After almost 20 years of intensive use by researchers all over the world, it is finally time to retire the old model and make a leap into the future of behavioral testing. The PhenoTyper 2 is ready to facilitate cutting-edge research for years to come.
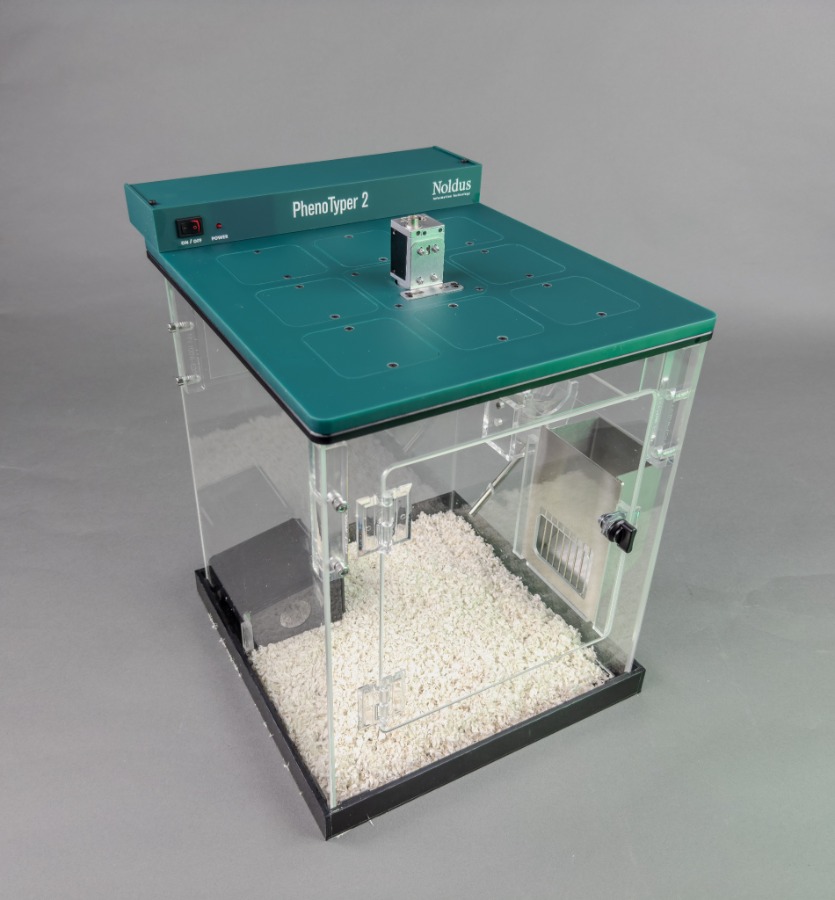